"Cloaked under the guise of objectivity, machine learning (ML) datasets are portrayed as impartial entities, giving the illusion of reflecting an “unbiased lookℽ at the world(Torralba & Efros, 2011). Yet, beneath this veneer, datasets are not neutral—they are infused with values, bearing the indelible imprints of social, political, and ethical ideologies woven into their fabric by their curators(Raji etal., 2021; Blili-Hamelin & Hancox-Li, 2023; Malevé, 2021).
This inherent value-laden nature becomes glaringly apparent in the perpetuation of social stereotypes and the stark underrepresentation of marginalized communities within the lifecycle of ML datasets(Wang etal., 2022; Buolamwini & Gebru, 2018; Zhao etal., 2021; Birhane etal., 2021; Denton etal., 2020). From inception to release, datasets emerge as political artifacts, etched with the signature of their creatorsℹ perspectives, organizational priorities, and the broader cultural zeitgeist, making them potent instruments in shaping narratives and reinforcing power structures(Winner, 2017; Hanna & Park, 2020; Birhane etal..."
Lire la suite
Position: Measure Dataset Diversity, Don’t Just Claim It
ARXIV, 28/04/2025
Partagé par :
Seddik Touaoula
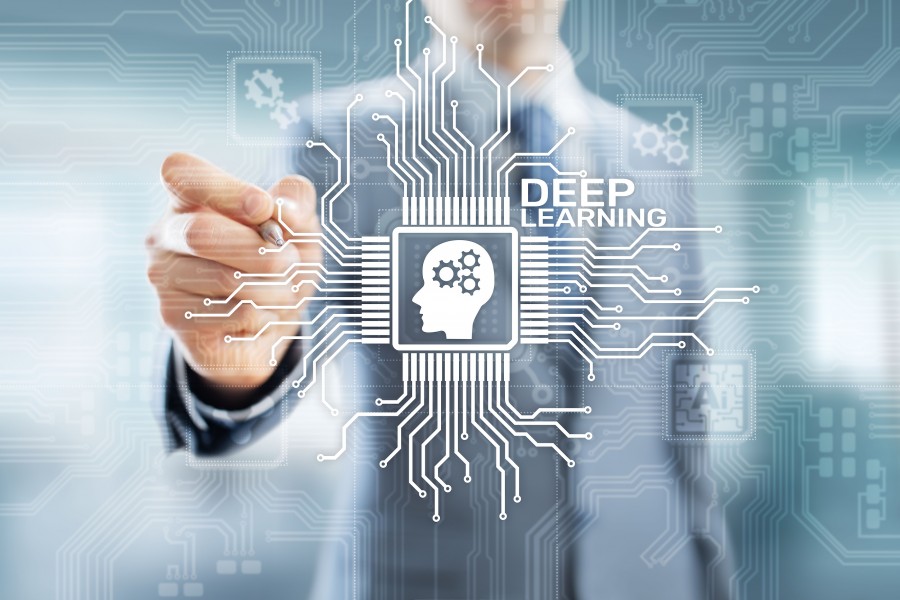
Informations liées
Thématiques
Définitions
Accueil Intelligence Artificielle
Machine Learning
Social
Fabricants de DM logiciels : ce que vous devez savoir
RUMB,
Partagé par : Beesens TEAM
En 2024, bannissons les termes "intelligence artificielle"
RADIOFRANCE,
Partagé par : Beesens TEAM
Metaverse and medical devices: what are the legal issues?
IBANET,
Partagé par : Équipe Beesens
Membres Premium A la une
Coach professionnelle certifiée / Formatrice
Fondatrice - KoKrea conseil
Human & IT - Collaboratif & réalité virtuelle
Associée KOS AVOCATS en e-santé