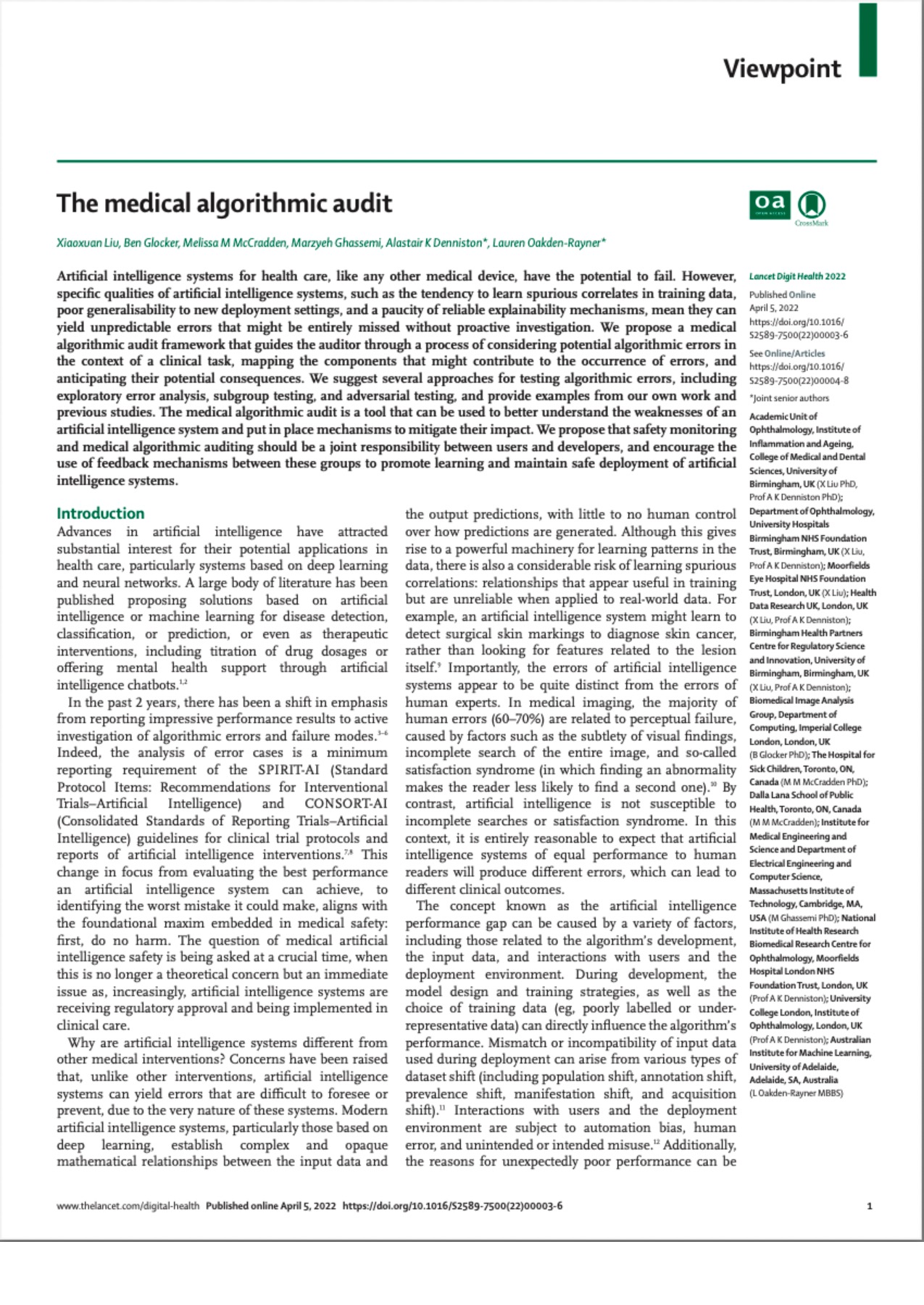
EN BREF ...
"Artificial intelligence systems for health care, like any other medical device, have the potential to fail. However, specific qualities of artificial intelligence systems, such as the tendency to learn spurious correlates in training data, poor generalisability to new deployment settings, and a paucity of reliable explainability mechanisms, mean they can yield unpredictable errors that might be entirely missed without proactive investigation. We propose a medical algorithmic audit framework that guides the auditor through a process of considering potential algorithmic errors in the context of a clinical task, mapping the components that might contribute to the occurrence of errors, and anticipating their potential consequences. We suggest several approaches for testing algorithmic errors, including exploratory error analysis, subgroup testing, and adversarial testing, and provide examples from our own work and previous studies." En bref issu de l'étude.
Rédacteur(s) de la fiche : Beesens TEAM
Introductio
1 - In tincidunt nunc ac velit tristique
- Pellentesque congue, magna elementum suscipit vestibulum
- Aenean eleifend sodales ipsum vitae consequat
- Quisque est leo tempus vel purus eu, placerat tincidunt nisl
2 - Sed lobortis elit vitae mollis consectetur
- In tincidunt nunc ac velit tristique
- Donec accumsan elit ac ornare eleifend
- Sed pellentesque suscipit quam ut finibus
- Fusce imperdiet neque sit amet ipsum ullamcorper scelerisque
3 - Lorem ipsum dolor sit amet
- Pellentesque congue, magna elementum suscipit vestibulum
- Aenean eleifend sodales ipsum vitae consequat
- Quisque est leo tempus vel purus eu, placerat tincidunt nisl
Conclusio
Pour accéder à ce contenu,
créez votre compte
gratuitement
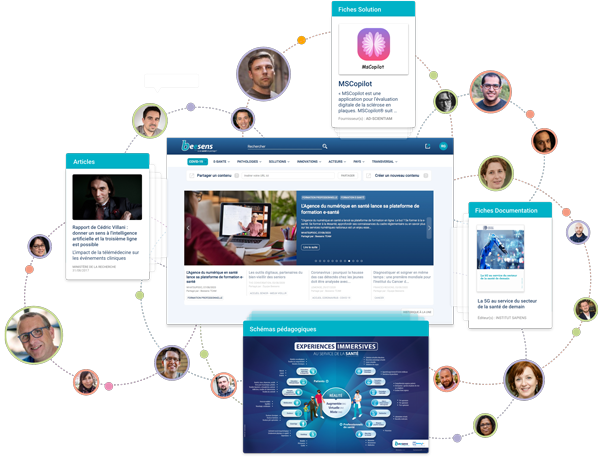
Accéder à :
- L'ensemble de la veille e-santé sélectionnée
par la communauté Beesens, - Des documents de références de la e-santé,
- Et bien plus encore...
Déjà inscrit ? Identifiez-vous