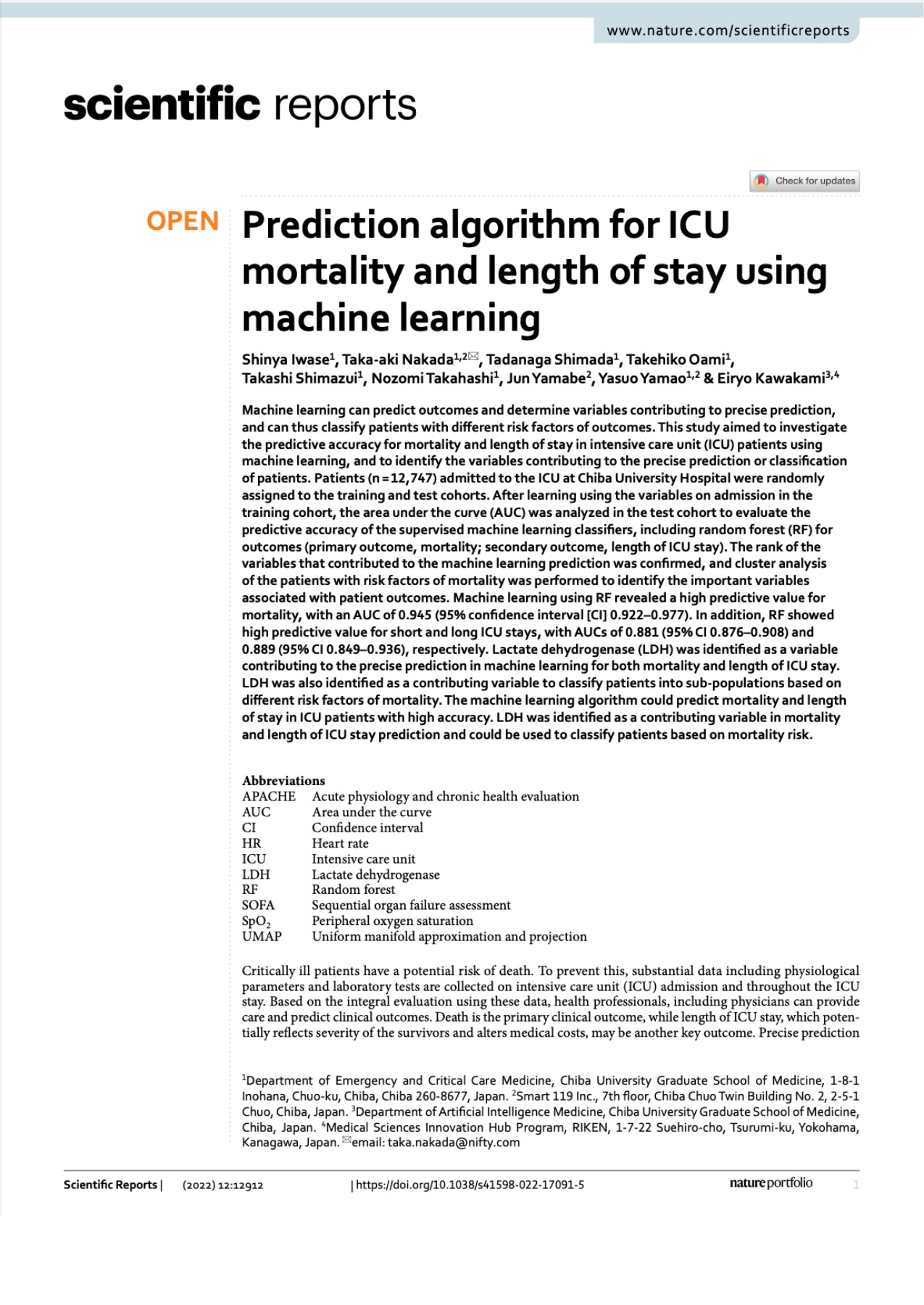
EN BREF ...
"Machine learning can predict outcomes and determine variables contributing to precise prediction, and can thus classify patients with different risk factors of outcomes. This study aimed to investigate the predictive accuracy for mortality and length of stay in intensive care unit (ICU) patients using machine learning, and to identify the variables contributing to the precise prediction or classification of patients. Patients (n = 12,747) admitted to the ICU at Chiba University Hospital were randomly assigned to the training and test cohorts. After learning using the variables on admission in the training cohort, the area under the curve (AUC) was analyzed in the test cohort to evaluate the predictive accuracy of the supervised machine learning classifiers, including random forest (RF) for outcomes (primary outcome, mortality; secondary outcome, length of ICU stay)." En bref issu de l'étude.
Rédacteur(s) de la fiche : Beesens TEAM
Introductio
1 - In tincidunt nunc ac velit tristique
- Pellentesque congue, magna elementum suscipit vestibulum
- Aenean eleifend sodales ipsum vitae consequat
- Quisque est leo tempus vel purus eu, placerat tincidunt nisl
2 - Sed lobortis elit vitae mollis consectetur
- In tincidunt nunc ac velit tristique
- Donec accumsan elit ac ornare eleifend
- Sed pellentesque suscipit quam ut finibus
- Fusce imperdiet neque sit amet ipsum ullamcorper scelerisque
3 - Lorem ipsum dolor sit amet
- Pellentesque congue, magna elementum suscipit vestibulum
- Aenean eleifend sodales ipsum vitae consequat
- Quisque est leo tempus vel purus eu, placerat tincidunt nisl
Conclusio
Pour accéder à ce contenu,
créez votre compte
gratuitement
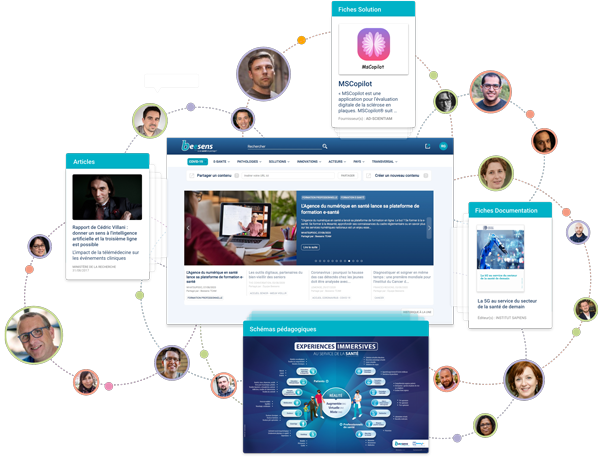
Accéder à :
- L'ensemble de la veille e-santé sélectionnée
par la communauté Beesens, - Des documents de références de la e-santé,
- Et bien plus encore...
Déjà inscrit ? Identifiez-vous