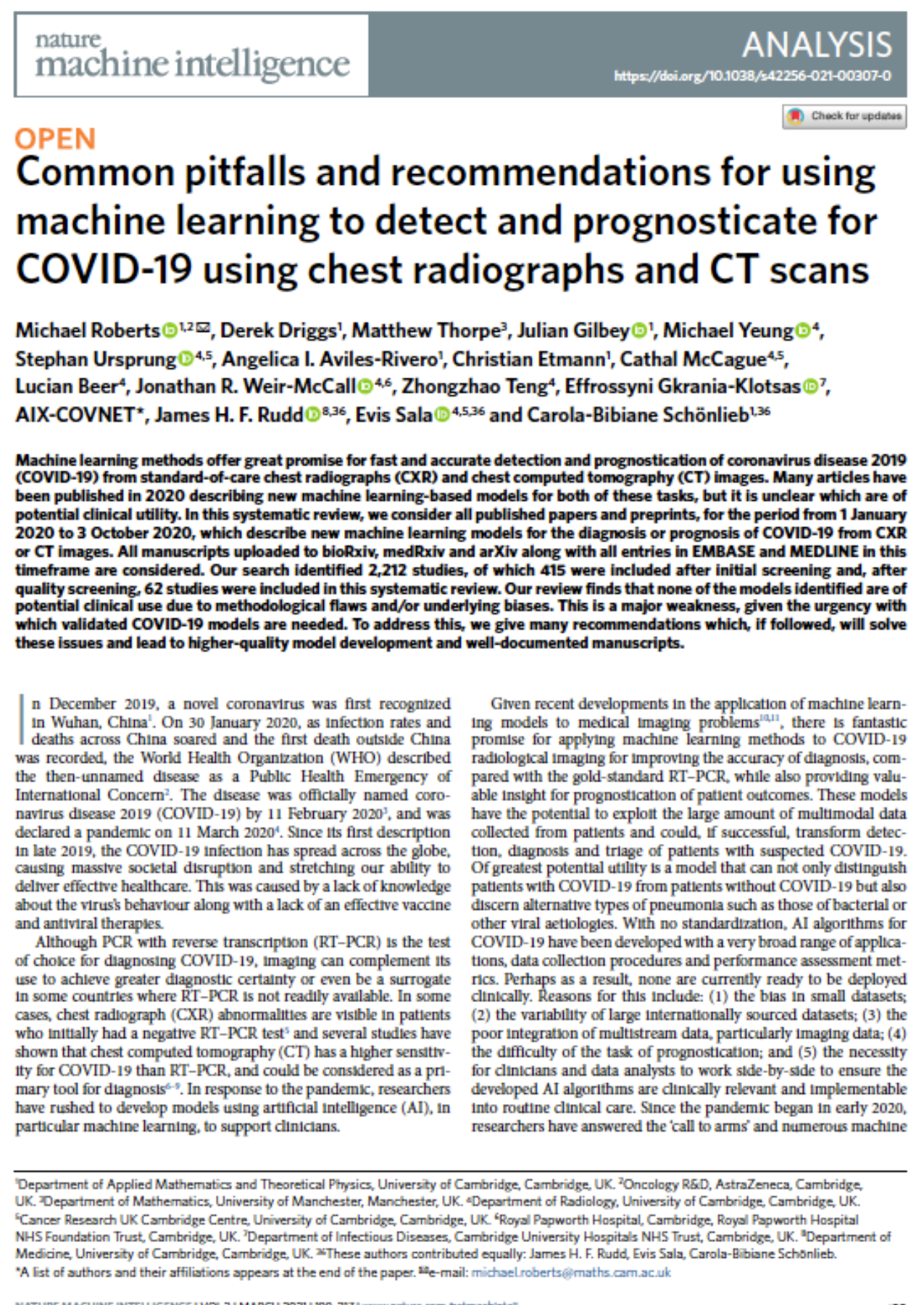
EN BREF ...
"Machine learning methods offer great promise for fast and accurate detection and prognostication of coronavirus disease 2019 (COVID-19) from standard-of-care chest radiographs (CXR) and chest computed tomography (CT) images. Many articles have been published in 2020 describing new machine learning-based models for both of these tasks, but it is unclear which are of potential clinical utility. In this systematic review, we consider all published papers and preprints, for the period from 1 January 2020 to 3 October 2020, which describe new machine learning models for the diagnosis or prognosis of COVID-19 from CXR or CT images. All manuscripts uploaded to bioRxiv, medRxiv and arXiv along with all entries in EMBASE and MEDLINE in this timeframe are considered. Our search identified 2,212 studies, of which 415 were included after initial screening and, after quality screening, 62 studies were included in this systematic review. Our review finds that none of the models identified are of potential clinical use due to methodological flaws and/or underlying biases. This is a major weakness, given the urgency with which validated COVID-19 models are needed. To address this, we give many recommendations which, if followed, will solve these issues and lead to higher-quality model development and well-documented manuscripts." En bref issu de l'étude.
Rédacteur(s) de la fiche : Beesens TEAM
Introductio
1 - In tincidunt nunc ac velit tristique
- Pellentesque congue, magna elementum suscipit vestibulum
- Aenean eleifend sodales ipsum vitae consequat
- Quisque est leo tempus vel purus eu, placerat tincidunt nisl
2 - Sed lobortis elit vitae mollis consectetur
- In tincidunt nunc ac velit tristique
- Donec accumsan elit ac ornare eleifend
- Sed pellentesque suscipit quam ut finibus
- Fusce imperdiet neque sit amet ipsum ullamcorper scelerisque
3 - Lorem ipsum dolor sit amet
- Pellentesque congue, magna elementum suscipit vestibulum
- Aenean eleifend sodales ipsum vitae consequat
- Quisque est leo tempus vel purus eu, placerat tincidunt nisl
Conclusio
Pour accéder à ce contenu,
créez votre compte
gratuitement
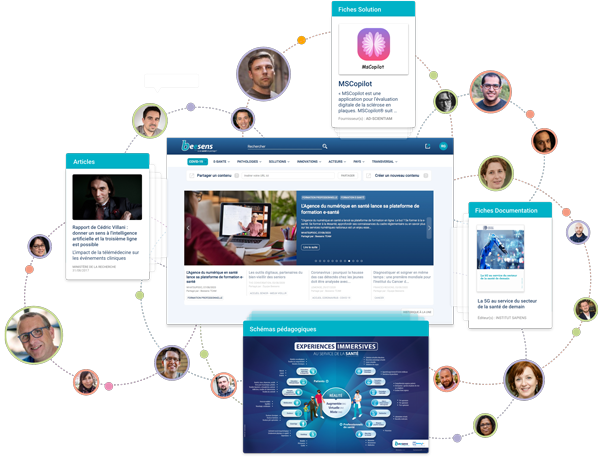
Accéder à :
- L'ensemble de la veille e-santé sélectionnée
par la communauté Beesens, - Des documents de références de la e-santé,
- Et bien plus encore...
Déjà inscrit ? Identifiez-vous