"The black-box nature of current artificial intelligence (AI) has caused some to question whether AI must be explainable to be used in high-stakes scenarios such as medicine. It has been argued that explainable AI will engender trust with the health-care workforce, provide transparency into the AI decision making process, and potentially mitigate various kinds of bias. In this Viewpoint, we argue that this argument represents a false hope for explainable AI and that current explainability methods are unlikely to achieve these goals for patient-level decision support. We provide an overview of current explainability techniques and highlight how various failure cases can cause problems for decision making for individual patients. In the absence of suitable explainability methods, we advocate for rigorous internal and external validation of AI models as a more direct means of achieving the goals often associated with explainability, and we caution against having explainability be a requirement for clinically deployed models.
Artificial intelligence (AI), powered by advances in machine learning, has made substantial progress across many areas of medicine in the past decade. Given the increasing ubiquity of AI techniques, a new challenge for medical AI is its so-called black-box nature, with decisions that seem opaque and inscrutable. In response to the uneasiness of working with black boxes, there is a growing chorus of clinicians, lawmakers, and researchers calling for explainable AI models for high-risk areas such as health care.
Although precise technical definitions of explainability lack consensus, many high-level, less precise definitions have been put forth by various stakeholders. For example, the General Data Protection Regulation laws in the EU state that all people have the right to “meaningful information about the logic behind automated decisions using their data”. Similar discussions have taken place in the clinical literature, in which it has been argued that clinicians might feel uncomfortable with black-box AI, leading to recommendations that AI should be explainable in a way that clinical users can understand. Indeed, Tonekaboni and colleagues report that surveyed clinicians “viewed explainability as a means of justifying their clinical decision-making”...."
Lire la suite
The false hope of current approaches to explainable artificial intelligence in health care
THELANCET, 01/11/2021
Partagé par :
Beesens TEAM
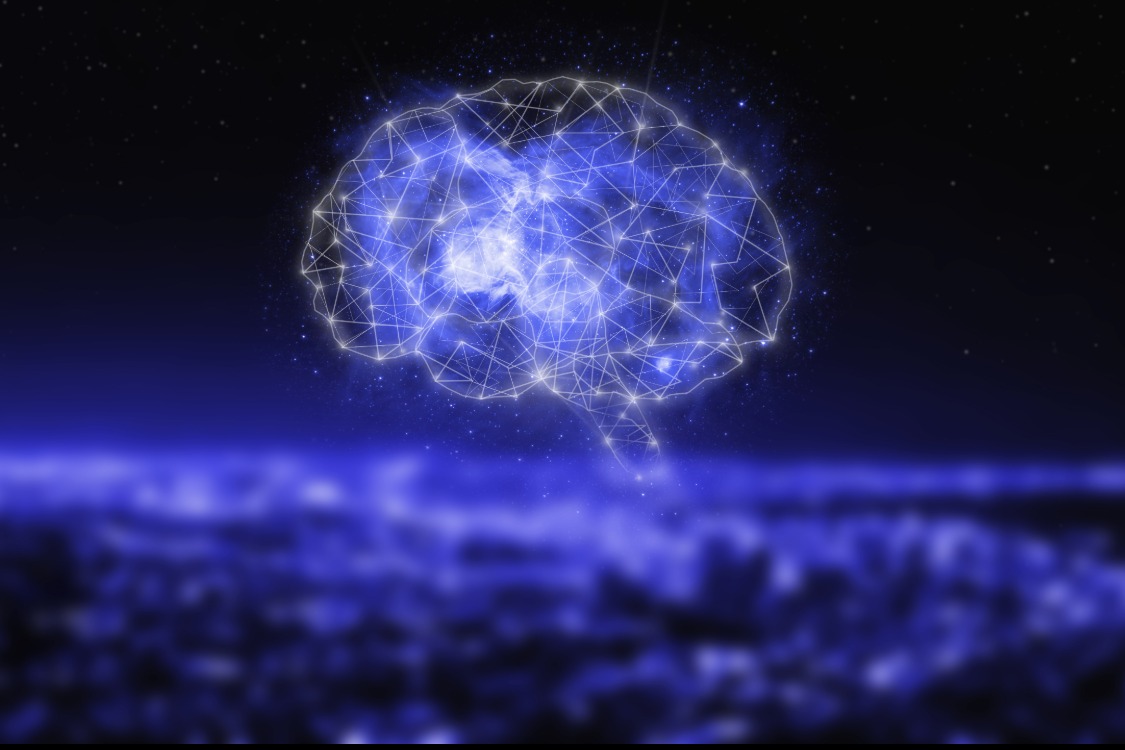