"PPrecision medicine has driven the high-throughput profiling of both molecular and medical imaging data to identify detailed tumor subtypes that better predict survival and treatment outcomes. Radiogenomic studies attempt to integrate two complementary data types to explain tumor imaging patterns using molecular information and vice versa. For example, radiogenomic studies have shown that image features [e.g., appearance of a tumor on computed tomography (CT) or magnetic resonance imaging] predict molecular patterns (e.g., gene expression, gene mutation, or molecular subtypes).1–6 Radiogenomic studies support the derivation of tumors’ biological states from noninvasive imaging and the correlation of molecular information and imaging phenotypes to better understand cancer heterogeneity. However, radiogenomic studies are often limited by the high dimensionality of the data, the simplifying model assumptions (e.g., linearity), and the lack of validation datasets.7,8
Deep learning techniques have been widely used on molecular and imaging datasets given their ability to handle high-dimensional inputs without feature engineering and to represent nonlinear and hierarchical relationships between model inputs and outputs. Several studies have used deep learning models such as convolutional neural networks, generative adversarial networks, and autoencoders to uncover radiogenomic associations.5,6,9,10 However, while these works report accurate predictions of imaging phenotypes from genomic data, they do not attempt to provide a biological interpretation of what the model has learned. While high classification accuracy is important, the ability to interrogate the model is critical to validating the learned radiogenomic associations..."
Lire la suite
Using deep neural networks and interpretability methods to identify gene expression patterns that predict radiomic features and histology in non-small cell lung cancer
TIC SANTÉ, 08/05/2021
Partagé par :
Beesens TEAM
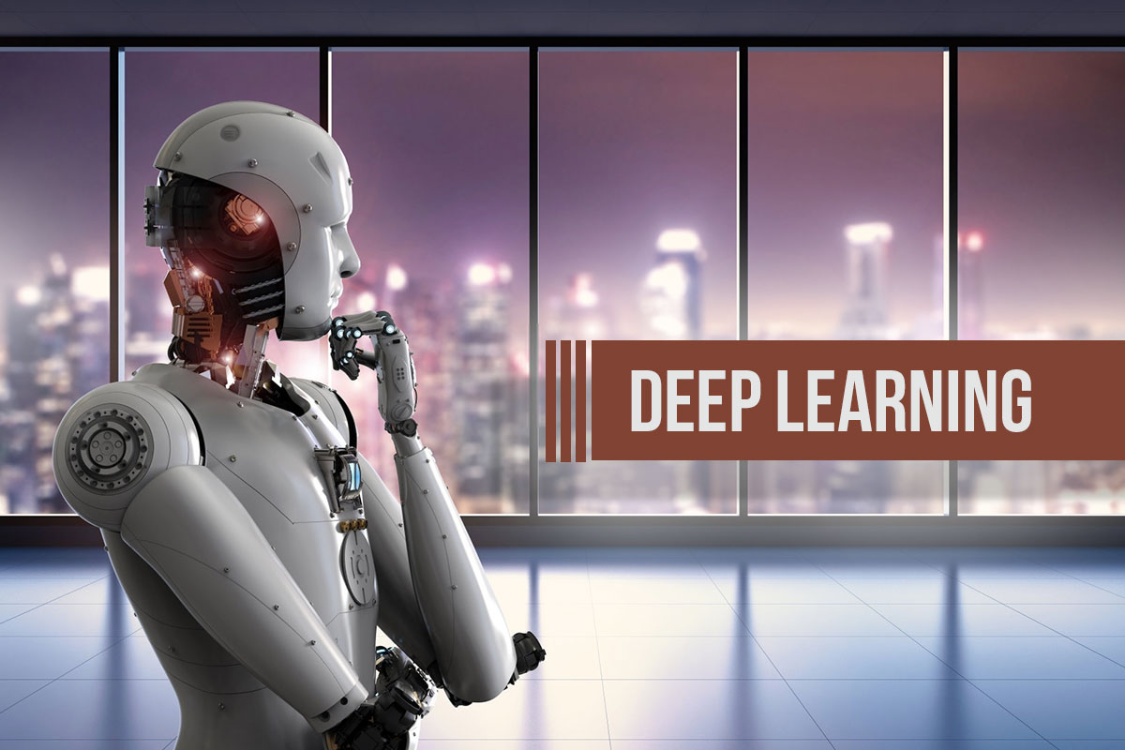
Informations liées
Thématiques
Accueil Intelligence Artificielle
Génétique
Cancer
Aide au diagnostic
Dépistage
Solutions
RPPS
Structures
ONI
Ordre national des infirmiers
The Path to Medical Superintelligence
MICROSOFT,
Partagé par : Awatif Taoufik
Ses préconisations relatives au DMP, son déploiement et son usage.
THINK TANK TÉLÉSANTE & NUMERIQUE EN SANTE,
Partagé par : Awatif Taoufik
IA générative : le risque de l’atrophie cognitive
POLYTECHNIQUE-INSIGHTS,
Partagé par : Awatif Taoufik
Membres Premium A la une
Coach professionnelle certifiée / Formatrice
Fondatrice - KoKrea conseil
Human & IT - Collaboratif & réalité virtuelle
Associée KOS AVOCATS en e-santé